They say, “an apple a day keeps the doctor away,” but in today’s data-driven world, maybe it should be “a dataset a day keeps inefficiency at bay.” The healthcare industry has long been perceived as a labyrinth of paperwork, patient files, and mysterious billing codes. Fortunately, we now live in an age where data analytics is stepping in as the superhero—cape and all—ready to rescue the medical field from its web of inefficiencies. But what exactly does data analytics do in healthcare, and why should you care? Well, let’s dive into this world where numbers have life-saving potential.
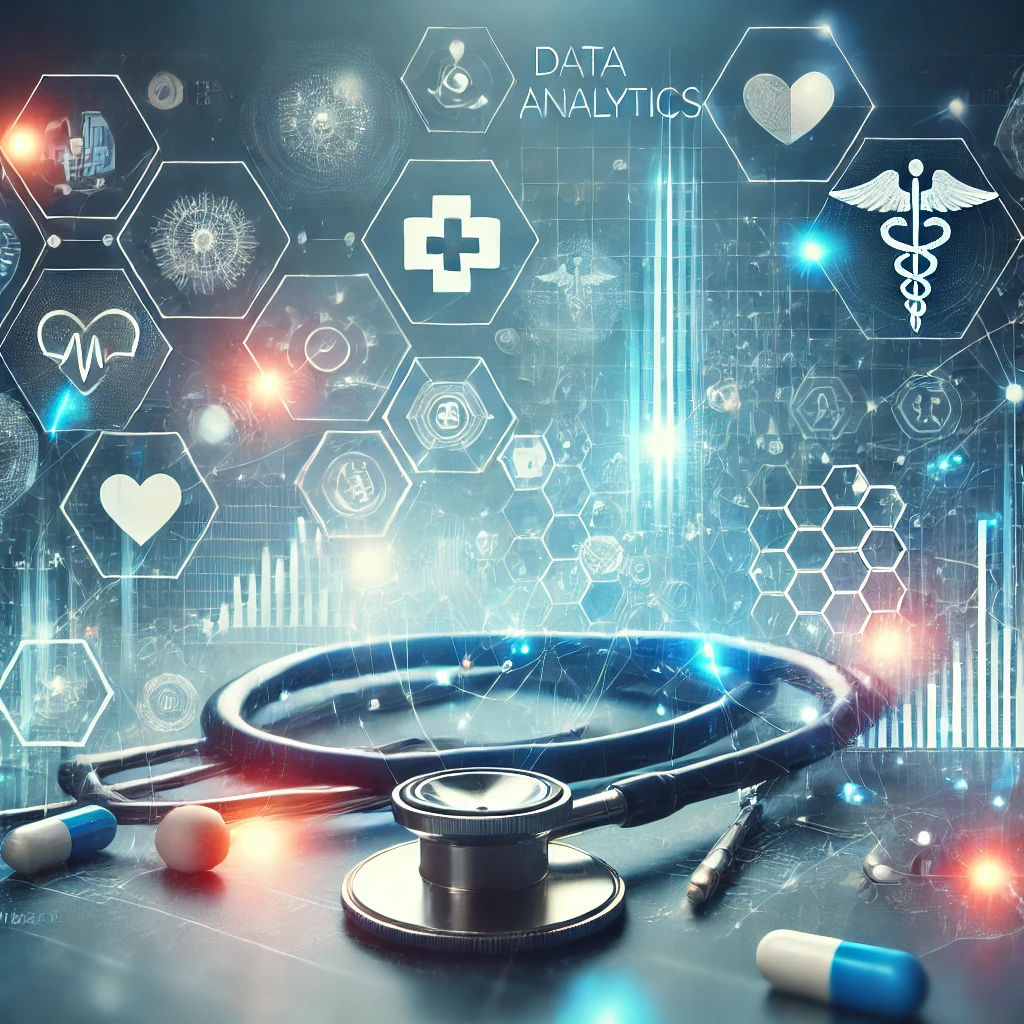
A Mess That Needs Fixing
Modern healthcare systems are, to put it mildly, a complex conundrum. From the ballooning costs of medical services to the challenge of keeping up with patient care demands, the system is often compared to a delicate balancing act on a unicycle—except the unicycle is on fire, and there’s a crowd watching. Hospitals and clinics are under immense pressure to streamline operations, improve patient outcomes, and somehow, in the process, keep costs down. Enter data analytics.
As technology advances, healthcare has become more dependent on data. Every visit to the doctor, every test result, and every insurance claim creates data. And, as this mountain of information grows, the healthcare sector is now realizing the importance of turning it into actionable insights.
Key Applications of Data Analytics in Healthcare: Saving Lives and Time
Improving Patient Outcomes
Data analytics is helping doctors move from being simply reactive to proactive—or, as we like to call it, from “I’ll see you when you’re sick” to “I’ll keep you from getting sick in the first place.” Predictive analytics can detect patterns in a patient’s data to forecast potential health issues, which leads to earlier interventions. And, when combined with wearables (yes, your Fitbit is watching), data analytics can track everything from blood pressure to heart rate, empowering physicians to tailor treatment plans to individual needs. It’s like having a crystal ball—but instead of predicting your future love life, it predicts your cholesterol levels.
Operational Efficiency: Because Time is Money
Hospitals are famously chaotic places—think of the waiting rooms alone, where time seems to stretch like a bad movie scene that never ends. Data analytics can optimize this experience by helping to streamline hospital operations. Analytics is being used to schedule staff, manage resources, and even reduce patient wait times, making sure that no one has to die of boredom before their actual treatment begins.
On a serious note, analytics-driven scheduling helps ensure that operating rooms and critical care units are used more efficiently, meaning fewer delays and happier patients (and doctors).
Fraud Detection and Prevention: Catching the Bad Guys
It’s no secret that healthcare fraud is a multi-billion-dollar problem. But who needs Sherlock Holmes when you have data analytics? By sifting through claims and billing data, analytics can uncover unusual patterns that may signal fraudulent activity. Think of it as healthcare’s very own “whodunit,” except the culprits are likely shady billing practices, not Professor Plum with the candlestick.
Population Health Management: Looking at the Big Picture
Data analytics can help public health officials and healthcare providers get the bigger picture. By analyzing data across populations, we can identify health trends, track outbreaks, and manage chronic diseases more effectively. This not only saves lives but also allows healthcare providers to focus their resources where they’re needed most.
The Role of Big Data and AI: It’s Like Healthcare, But on Steroids
If regular data analytics is impressive, then big data is the supercharged version. Big data allows us to analyze vast and diverse datasets that, once upon a time, would have caused even the most patient scientist to throw in the towel. These massive datasets can include everything from genomic data to social determinants of health, and when combined with artificial intelligence (AI), the possibilities are endless.
AI is already revolutionizing areas like diagnostics and medical imaging, where it can identify abnormalities in X-rays or MRI scans faster—and often more accurately—than human eyes. In drug discovery, AI is accelerating the process by identifying promising compounds much more quickly than traditional methods. It’s like having a speed-dating service for pharmaceuticals: only the best matches make it to the next round.
Challenges in Implementing Data Analytics in Healthcare: It’s Not All Sunshine and Rainbows
Of course, nothing comes without its share of hiccups. Data analytics in healthcare faces significant challenges—chief among them, privacy and security. HIPAA regulations are no joke, and ensuring that patient data is kept secure is paramount. If there’s one thing you don’t want happening, it’s your medical records being mixed up with your Netflix viewing history.
There’s also the matter of integration. Many hospitals and healthcare systems use different electronic medical record (EMR) systems, which can make it difficult to share and analyze data across platforms. And let’s not forget that healthcare professionals need to be data-literate to fully utilize these tools. After all, you can have all the data in the world, but it won’t do you much good if no one knows how to read it.
The Future of Data Analytics in Healthcare: Looking Ahead
Looking ahead, the future of data analytics in healthcare is brimming with potential. Imagine a world where predictive models can foresee disease outbreaks, not months or weeks in advance, but days—even hours—before they happen. Or, where AI-driven tools become the norm in everyday care, making healthcare more efficient and accessible for everyone.
But no matter how much technology advances, one thing will always remain the same: the human element. Behind every dataset is a patient, a person with a story. And as data analytics continues to evolve, its true power lies not just in crunching numbers, but in helping healthcare providers deliver the best care possible.
Conclusion: Data, Doctors, and the Future
So, there you have it. Data analytics is quickly becoming the lifeblood of modern healthcare, streamlining operations, improving outcomes, and, most importantly, helping people. Who knew that a bunch of numbers could do so much? In a world where a single data point can mean the difference between life and death, one thing’s for sure: healthcare will never be the same again. And if you’re ever feeling under the weather, just remember—there’s probably a dataset for that.